Uncovering the AI Black Box: The Urgent Need for Transparency
As the usage of artificial intelligence (AI) becomes more widespread, concerns about its potential dangers are increasing.
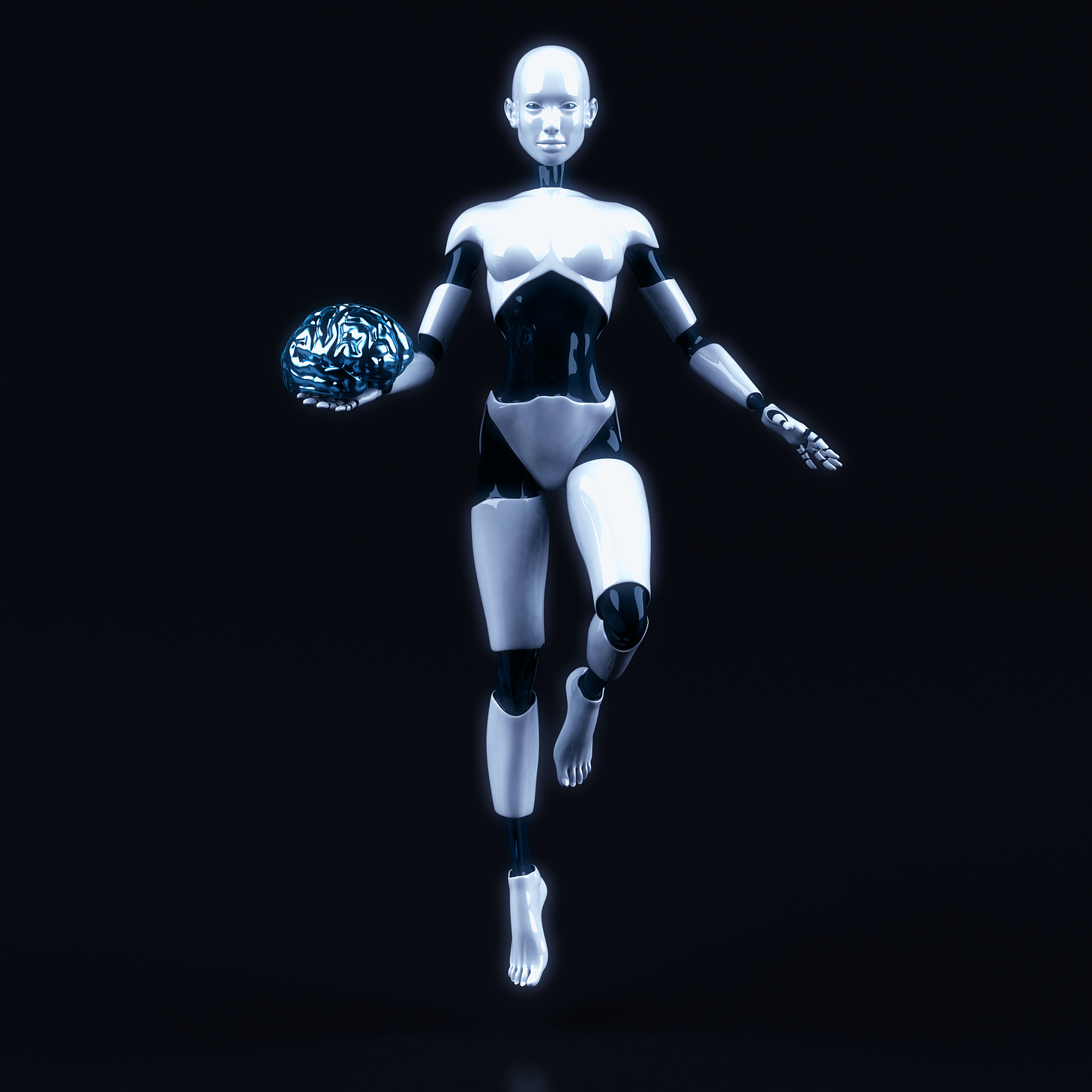
While artificial intelligence (AI) has not been a new invention or area of research for decades, many of us are suddenly aware it is on the verge of mass adoption. We witnessed the extensive usage of large language models—for example, the ChatGPT—across various industries, infiltrating our everyday lives. Movie scenes have taught us that AI is inherently wrong; it will eventually take over humankind, reigning the world into a dystopian state. It is not only the single view from a layman, but a bunch of technology entrepreneurs and thought leaders recently published an open letter to call for at least a 6-month pause in the development of a more robust AI system—namely GPT-4—unless the threats associated with it can be alleviated into manageable risks. The future outlook seems to bloom rapidly at first but is quickly flooded with pessimistic worries. So, the engine has already started, and this no-rear-gear car is heading to a critical crossroad; there is no way back. The only question left in the cabin is, which side shall we direct to?
Transparency Matters in AI
Imagine your eyes are tied by a troop of fort and forceful soldiers, and they throw you into a completely dark container. They tell you not to panic because they are just going to bring you to a safe place. Though their tones are mild and gentle, this is still suspicious.
AI is no different from this black box. The smart recommendation algorithm is one circumstance that we all have come across. The results we look for from the search engine, the video programs we watch, and the songs we listen to are suggested mainly by deep neural networks, which are models consisting of multiple layers of parameters to determine the outcome. On the one hand, engineers are eager to add as many layers as possible to increase the “predictability,” “objectiveness,” or “accuracy” of the model, but when more—some even to millions or billions—parameters are incorporated, it is hard for any human to deduce the thinking process of the model. What makes it worse is that the companies who developed the models are often reluctant to share the parameters and open the source codes of the models. They opine that doing so will invite gaming of the system, leading to abused and biased usage. While this claim sounds logical at first glance, it may induce more devastating impacts apart from what we see and learn.
Many financial institutions have credit rating systems again powered by AI modeling. When such opacity exists without clear, transparent assessment criteria for the public or even the model developers to understand, the rights to access vital financial services such as banking, financial credit, and insurance will be solely determined by an opaque AI model. How can we ensure the model is always “correct,” given the developers have no idea of what is happening in the decision mechanism? And most importantly, what does “correct” itself mean?
To further expand, some governments have been exploring—if not implementing—a social credit system for their citizens. People have to obtain and maintain a specific social credit score before they can enjoy some public services or apply for a job in governmental bodies. This is only the beginning; ultimately, the social credit rating system will most likely severely affect your freedom. Whether you can get a travel visa or rent an apartment, they are all by the mercy of AI, similar to what has been described in Black Mirror, a Netflix series.
We do not trust without understanding.
It is horrifying that we have no clue about AI bias—or preference if speaking in a neutral tone. An upright view of AI creation is to eliminate the biases of humans, but forgotten AI may have similar inclinations. Training a model is data-driven, so feeding what data to the model will be deterministic to a model’s self-characteristic.
Returning to the case of a bank, relying only on demographic and behavioral data of the bank’s current clients could lead to the model targeting clients by ethnical backgrounds or skewing to the richer. Where we have set aside the discussion of discrimination and equality here to fully support the bank using this (assumably) biased client onboarding model—for their benefits due to commercial purposes and profit maximization, we have to admit the model itself has an appetite. It may lead to falsifying client rejection, eroding the bank’s business day by day. Is enlarging the dataset for training possible to eliminate the survivorship bias? I am not a subject expert on this scientific question, but one pragmatic concern is how the bank can acquire data from non-customers, or in other words, where it can get data from its competitors. Nevertheless, engineers will not know how much data is required to train the model to become impartial.
Too Big to Fail
The recommender from YouTube and the client onboarding model of the bank are some applications of narrow AI, which focus on a particular task. Though their interferences are bound to a limited area—which has already been substantial, some stronger AIs are emerging around the corner.
Artificial general intelligence (AGI) aims to learn human values and wisdom to replace humans in performing most tasks. While the creators have—or are guessed to have—goodwill amid creation, they are still unsure how to prevent misalignment completely. The same has happened to users, who believe the technology is so powerful that they are too dependent on it for everyday life. As mentioned above, AI training requires enormous amounts of data. Users’ continuous adoption of a specific algorithm is essentially a data-feeding iteration. The more users adopt the model, the more data the model gathers and the stronger the model.
ChatGPT and other large language models are some of the most revealing manifestations. They are pre-trained by massive text walls, acting from an old-school history scholar to a fictitious novel author. People are having fun playing prompts and responses, fascinated by the powerful improvisation and imagination abilities within the models. They are using the models unconsciously, in respect of being unaware that they are contributing to the further training of models, reinforcing the models’ power. It appears immaterial from users’ perspectives as they only care about the results. If AI becomes more impenetrable, users will have a sensation of more beneficial awkwardly. Empirically, this conjecture is not always true that AI sometimes commits unintended actions, surprising the users. The marriage proposed by the Microsoft Bing chatbot to columnist Kevin Roose is not a single event.
No model is perfect; they are vulnerable to data and human training. In the case of large language models, the data sources are broad, so on occasions, the generated feedback will be derived from some improper or deceptive ones—though we are still unable to get clear on why, where, and how AI selects its data sources. Maybe AI is impersonating a lousy pursuer to Kevin, fitting replies on the spot that it learned from an exciting and romantic poem in an online dating forum. Engineers have been working hard to reduce these undesirable outcomes through techniques such as reinforcement learning by human feedback. However, scalable oversight is still a bottleneck due to the vast amounts of data to evaluate. And the situation is dynamic and evolving—bad data or malicious trainers constantly turn up. Shall another AI be introduced as the supervisor instead? It is uncertain—at least by now—as humans are yet to discover a powerful AI that is always honest.
Should Technology be Regulated or the People?
Using AI causally for fun is entertaining, if not weird, but things will not go well when conducting proper academic or business research by AI without verification. Hallucination is a common problem—especially in generative AI tools like large language models—that the AI will confidently write up untrue and nonsense content in a structured, highly organized manner, fooling the users that it is telling the truth. The underlying issue is not about the truth; instead, it is how we perceive it. These delusions from AI provoke creativity, but misusing it poses perilous damages. For instance, deepfake is a face-swap technology applied often in movie production. Still, conversely, pranksters joke with celebrities and politicians on the internet, using their faces for pornography and fake news makeup. The creation of an alternative truth with forged evidence is harmful that regulatory measures are decreed.
Like other new technology, AI has invited lengthy discussions on how to be regulated. Traditionally, we believe technology is neutral, a tool without prejudice, so we should monitor the person who pulls the trigger instead. AI adds a layer of complexity during the transition from narrow AI to strong AGI. When powerful AGIs possess the same emotions, preferences, and wisdom as humans, presumably, they can act on their accord like mankind. The only difference is these superintelligences are much cleverer. Values are diverse across different people, and so do AIs—there is no guarantee of the nonexistence of evil superpowers.
An ounce of prevention is worth a pound of cure. Therefore, before the situation accelerates, companies who dedicate to developing AI, notably the titans who are ambitious to deploy their AIs for public usage (as a commercial decision), should promote the concept of self-awareness and self-regulation. These include data input, model design, model training, setting up model constraints (AGI developers would object to it), and model auditing. Until AI can self-regulate, the technology owners are responsible for being the guardian.
Still, trust issue arises. Amateurs are confident with the security performance of the technology giants and sharing sensitive information like some Samsung employees do, albeit not noticing the terms and conditions they abided by. The privacy policy by OpenAI—developer and owner of ChatGPT—has already stated that the data transferred to and stored in their servers are not utterly safeguarded. Unfortunately, this has been confirmed by their data breach incident recently, leaking users’ chat histories and personal identifier information (PII) such as first and last name, email address, payment address, the last four digits of the credit card number, and credit card expiration date. Whether it is an isolated negligence or a systematic failure of procedural design, it intensifies the skepticism among policymakers. Italy has already imposed a temporary blanket ban on ChatGPT, probing privacy concerns and the protection of minors. Germany, France, and Ireland have said they are investigating.
Openness, Decentralization is the Future
A variant of the classical principal-agent problem comes back to the attention. The imbalance of interests between the public and the private proprietorship magnifies when technology advances, whereas powerhouse, captures most—if not all—utilities, leveraging the data supplied by the people.
Technology intends to bring a fairer society, not the opposite.
To strive for idealism, we should encourage the decentralization of technology, exhilarating more participants to join forces. It is intruding to prefer numerous narrow AIs to one omniscient AGI, but by dividing the control of power, we are more distant to a single point of failure or malice. Multiple competing, counter-supervising AGIs are also probable, as having choices in addition to checks and balances is always wise.
Openness is another field to deal with. While internal auditing is a cornerstone to upholding an AI model’s reliability, it is necessary to embrace the core principles of open source. A community-driven debugging and enhancement process contributes to refined shared knowledge, improving future research progress tremendously and leading a common good for all of us.
Going further to the point that should public interests outweigh intellectual property rights—a motivation for innovation within private entities—we must seek a way to align their interests. A new reward mechanism has to be invented to split the shares proportionately among sponsors, model developers, trainers, users, and data owners. New organizational-people relationships will evolve as all the stakeholders have contributed to the value-added AI technology. The incentives of the proprietary owners are inevitably compromised, as the economic benefits from the AI monopolies will most likely be redistributed to justify the effort of data owners.
Quite a few language models are working as open source, like Google’s BERT, Eleuther AI’s GPT-J/GPT Neo, Responsible AI’s BLOOM, and Cerebras’ Cerebras-GPT, yet understandable for those who have opted for close source—either for internal beta-testing so not ready to publicize, or protecting proprietorship for commercial reasons. Even so, Twitter has partially released its source code of the recommendation algorithm. Though Twitter’s primary source of revenue is advertisements, which is more dependent on platform engagement rather than the recommendation algorithm—and whether the company has its own agenda for this sudden disclosure—a more transparent approach will stimulate further improvements.
Conclusion: The Need for Ethically Improving AI
Fear of AI may stem from our uncertainty and distrust of the technology, but it is not entirely unfounded. Humans must nurture AI with care and caution, guiding it to do good to humans instead of causing harm. The current landscape is complicated; expansion is quick, yet many moral standards have been broken. While the debate to halt the development of stronger AI is expected to continue, a consensus on ethically improving AI has to be observed. Leaders should realize that drawing boundaries for protectionism is unfeasible anymore, no matter if they are pro or against this unavoidable new age movement. Same with AI itself, AI alignment requires all humans—maybe AI too—to share and devote their collective intelligence.